Introduction
The first coronavirus disease 2019 (COVID-19) case in Turkey was reported on March 11, 2020 (1). The number of confirmed cases with symptoms increased afterward, reaching 5000 per day by mid-April (2). With the effect of mitigation measures, the number of daily cases started to decline, and by June, there were about 1000 cases per day. This number was stable up until November when the second wave started. İstanbul, a metropolis of approximately 16 million inhabitants, was the epicenter of COVID-19 in Turkey.
In Turkey, the pandemic response was led by a scientific council formed by the government (3). Hospitals acted according to the regularly updated guidelines published by the scientific council for the diagnosis and treatment of COVID-19 patients (4). At the beginning of the pandemic, admission to the hospital was recommended for patients over the age of 50 years and those with comorbid conditions, severe pneumonia, or laboratory values indicative of a worse prognosis.
Much has been learned about the clinical features of COVID-19 during the past two years of the pandemic (5). The early reports indicated that COVID-19 could progress differently among infected individuals, ranging from asymptomatic carriage to respiratory failure leading to death (6). In a report of 72,314 cases from China, the spectrum of disease varied from mild (81%) and severe (14%) to critical (5%) (7). In a systemic review and meta-analysis, the all-cause mortality rate of hospitalized COVID-19 patients was 10%, with a predictive interval of 2% to 39% (8). The authors concluded that substantial between-study heterogeneity led to a large 95% predictive interval suggesting high uncertainty. Clearly, high-quality cohort studies with defined severity criteria and adequate follow-up times are needed (9, 10). It is important to determine critical illness outcomes and associated factors, which are vital to determine hospitalization criteria, which in turn will affect medical resource allocation (11).
Here, we describe the demographic characteristics, clinical features, 28-day critical illness development, and factors associated with critical illness among COVID-19 patients treated at the beginning of the pandemic in one of the main pandemic hospitals in Istanbul, Turkey.
Materials and Methods
Study Setting and Design
We conducted a single-center, prospective cohort study. All hospitalized ≥18-year-old patients with confirmed COVID-19, i.e., positive RT-PCR assay for the SARS-CoV-2 genome detected in a nasopharyngeal or oropharyngeal swab specimen, were included. The study period started with the first confirmed patient in our hospital on March 12, 2020, and included patients hospitalized until May 12, 2020. Testing was performed on patients who fulfilled the criteria for possible COVID-19 according to the Turkish COVID-19 Guideline (4). Essentially, patients whose symptoms and signs were compatible with viral pneumonia and some cases based on clinical judgment were tested. Admitting a suspected COVID-19 patient to the hospital was mostly a clinical decision based on age, presence of comorbidity, dyspnea, hypoxia, and extent of pulmonary involvement detected with thoracic computed tomography (CT).
Study Participants
There were 521 patients hospitalized within the study period. The patients (n=55) who were transferred to or from our center were included in the study, provided that their medical records could be retrieved from other centers. As the outcome was the development of critical illness, we excluded patients who had invasive mechanical ventilation or died within 24 hours of admission (n=41) (Figure 1). In addition, healthcare-associated COVID-19 cases were excluded (n=3). After these exclusions, 477 patients were eligible and followed up for a period of 28 days postadmission.
The Ethics Committee of Marmara University School of Medicine approved the study on June 2020, with the decision number 09.2020.572. The requirement for written informed consent was waived by the board due to the urgent need to collect data. We followed the Strengthening Reporting of Observational Studies in Epidemiology (STROBE) guidelines.
Data Collection
Demographic information, exposure history, comorbidities, comedications, symptoms, signs, imaging and PCR results, vital signs and clinical course including medications, response to treatments, laboratory parameters, intensive care unit (ICU) referral, and prognosis were prospectively collected in forms standardized for COVID-19 patients. This information was then transferred to an electronic database, after which four physicians (US, PA, AT, HB) went through the data extensively, cross-checked the authenticity, and completed missing information using archival and electronic records.
Definition of the Variables
The outcome was progression to critical illness, either invasive mechanical ventilation or death within 28 days postadmission. Patients were reached by phone call to determine their readmission status, and their survival status was checked from a centralized electronic death registration system.
The patients’ illness severity on the day of hospitalization was defined by the World Health Organization (WHO) ordinal scale for clinical improvement (12). It is an 8-point ordinal scale including 0, uninfected; 1, ambulatory with no limitation of activities; 2, ambulatory with limitation of activities; 3, hospitalized, mild disease, no oxygen therapy; 4, hospitalized, mild disease, oxygen by mask or nasal prongs; 5, hospitalized, severe disease, noninvasive ventilation or high-flow oxygen; 6, hospitalized, severe disease, intubation and mechanical ventilation; 7, hospitalized, severe disease, ventilation and additional organ support such as renal replacement therapy or extracorporeal membrane oxygenation; and 8, death. Although the minimum scale for hospitalization was 3, we had some patients with a score of 2 who could have been followed in an ambulatory setting.
Age, sex, smoking status, body mass index (BMI), number of comorbidities, vital signs, laboratory findings, and period of admission to the hospital were the independent variables. The worst recorded value within the first 24 hours of hospitalization was recorded for vital signs. The quick sepsis-related organ dysfunction assessment (qSOFA) score was also calculated (13). The first available laboratory findings within 72 hours of hospital admission were noted. The hospital admission period was categorized as two weekly periods within the study time.
Statistical Analyses
Descriptive statistics are presented as percentages and medians (interquartile ranges [IQRs]). Categorical variables were compared with the chi-square and Fisher’s exact tests. Continuous variables for two independent groups were analyzed by the Mann-Whitney U test. Multivariable analysis was carried out with 451 (94.5%) out of 477 patients because of missing data. The Cox proportional hazards model with stepwise backward elimination was used, and the strengths of associations are presented as hazard ratios (HRs). Incidence rates and HRs were presented with 95% confidence intervals (CIs). The concordance index (C-index) was calculated. The statistical significance was set as p<0.05.
Results
Demographics and Characteristics
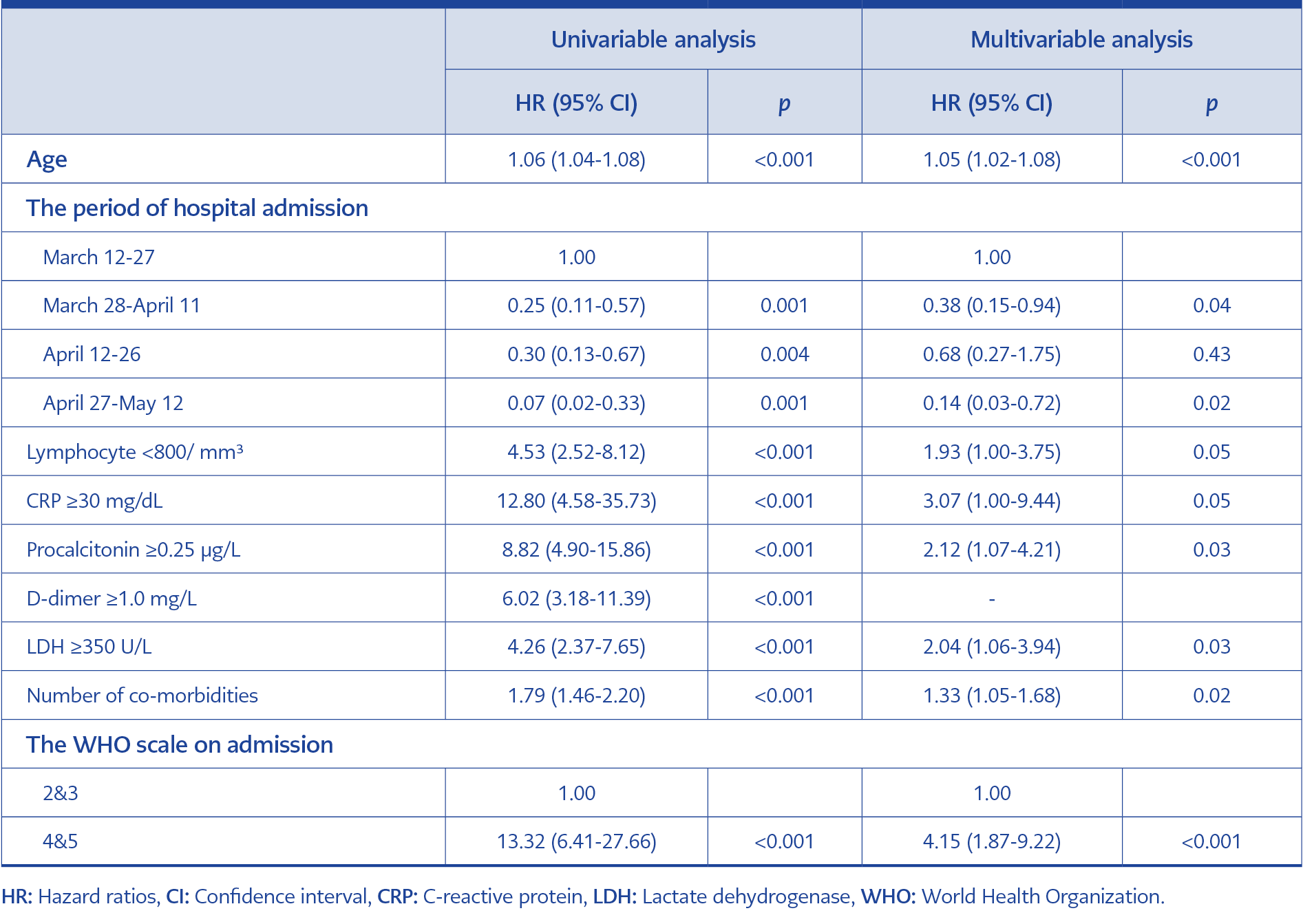
Table 1. Cox regression analysis for the association between clinical characteristics and progression to critical illness within 28 days of follow-up.
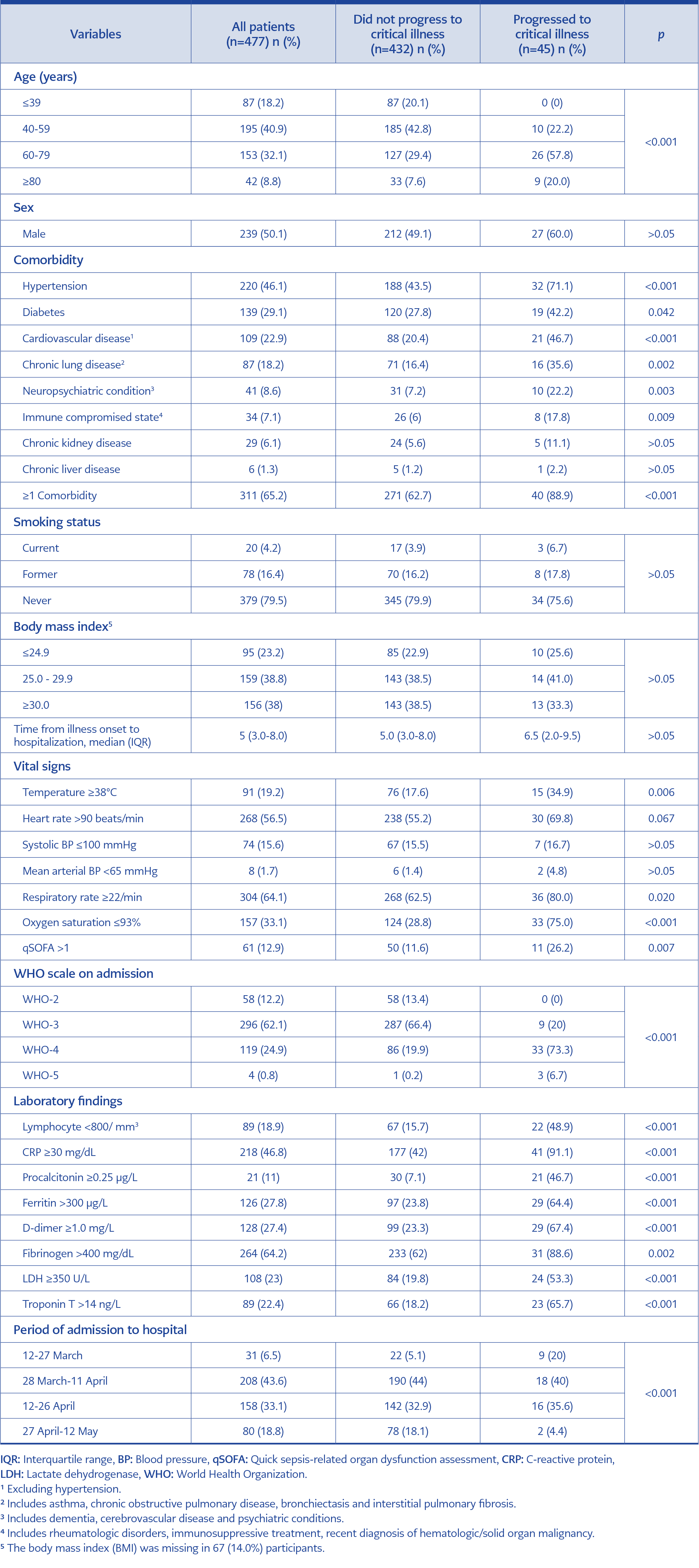
Table 3. Clinical characteristics on admission associated with progression to critical illness within 28 days of follow-up.
Overall, 477 patients hospitalized with confirmed COVID-19 were included in the analysis. The median (IQR) age of the cohort was 55.0 (44.0-67.0) years, and 239 (50.1%) were male.
The most common presenting symptom was cough followed by dyspnea, fatigue, myalgia, fever, and headache (Table 1). Sociodemographic variables, comorbidities, smoking status, BMI, vital signs, laboratory findings, and the period of hospital admission are presented in Table 2. On admission, 354 (74.2%) cases were classified on a scale of 2-3, and 123 (25.8%) were classified on a scale of 4-5 according to the WHO scale (Table 2).
The Outcomes
Among the 477 patients, 61 (12.8%, 95% CI=10.0%-16.1%) were admitted to the ICU. Forty patients (8.4%, 95% CI=6.1%-11.2%) required mechanical ventilation. Thirty-four (7.1%, 95% CI=5.0%-9.8%) patients died within the 28-day follow-up (Figure 1). Overall, 45 (9.4%, 95% CI=7.0%-12.4%) patients developed critical illness. The median (IQR) duration from hospital admission to invasive mechanical ventilation and death was 5.0 (3.0-8.8) and 12.0 (8.0-20.0) days, respectively. The median (IQR) hospital length of stay was 7.0 (5.0-11.0) days.
Treatment Characteristics
Four hundred seventy-three (99.2%) patients were given hydroxychloroquine alone, and 199 (41.7%) patients were given hydroxychloroquine combined with azithromycin. Favipiravir (n=147, 30.8%) was administered in patients with peripheral oxygen saturation ≤93% in ambient air. Oseltamivir was coadministered to 31 (6.5%) patients. Corticosteroids were given to 46 (9.6%) patients and tocilizumab to 30 (6.3%). Anticoagulant thromboprophylaxis with low-molecular-weight heparin (LMWH) was given to 295 (61.8%) patients. While 14 (45.2%) patients were given LMWH prophylaxis in the first two weeks of the study period, this number increased to 62 (77.5%) in the last two weeks (p<0.001).
Factors Associated with Critical Outcome
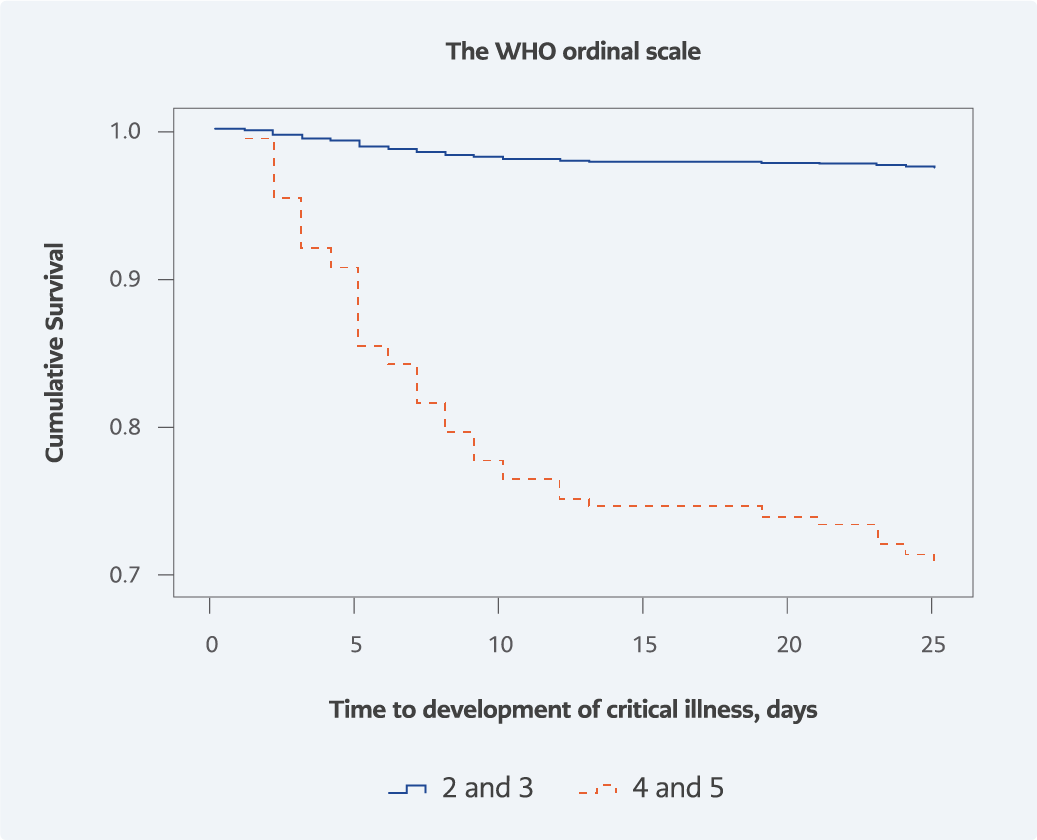
Figure 2. Time to development of critical illness by the baseline WHO
ordinal scale for clinical improvement.
The WHO scale for clinical improvement showed a significant association with the progression to critical illness. While 29.3% of patients on a scale of 4-5 on admission progressed to critical illness, this rate was 2.5% for those on a scale of 2-3 (p<0.001) (Figure 2). The rate of critical illness decreased significantly as the pandemic advanced. While 29.0% of patients who were hospitalized during the first two weeks of the pandemic progressed to critical illness, this rate was 2.5% for patients hospitalized during the last two weeks of the study period (p<0.001) (Figure 3).
Univariable and multivariable analyses for progression to critical illness within the 28-day follow-up are presented in Table 3. Age, sex, number of comorbidities, the WHO scale for clinical improvement, baseline laboratory values, and period of hospital admission were included in the multivariable model. Age was significantly associated with progression to critical illness; each year had an HR of 1.05 (95% CI=1.02-1.08). The number of comorbidities was also independently associated with critical illness (HR=1.33, 95% CI=1.05-1.68). Among the laboratory values, procalcitonin (PCT) ≥0.25 µg/L (HR=2.12, 95% CI=1.07-4.21) and lactate dehydrogenase (LDH) ≥350 U/L (HR=2.04, 95% CI=1.06-3.94) were independently associated with critical illness. Patients on a scale of 4-5 on admission had an HR of 4.15 (95% CI=1.87-9.22) for progression to critical illness compared to those on a scale of 2-3. The risk of critical illness decreased significantly as the hospitalization period advanced. Compared to the first period, the HR was 0.14 (95% CI=0.03-0.72) in patients hospitalized during the last two weeks. The C-index of the model was 0.92.
Discussion
This study describes the demographic characteristics, clinical features, and prognosis of 477 COVID-19 patients followed by a university hospital in İstanbul from mid-March to mid-May. The median age was 55.0 years (IQR=44.0-67.0), with an equal distribution of sexes. The 28-day mortality rate was 7.1%. The rate of progression to critical illness was 9.4%.
Some studies carried out at the beginning of the pandemic with comparable characteristics of participants reported similar rates for the development of critical illness or death. For example, a cohort study from China, including patients with a mean age of 46.1 years, reported a rate of 8% for the development of adverse outcomes (14). Another study from two hospitals in New York City, including patients with a median age of 62.2 years, revealed a mortality rate of 10.2% (15). However, comparing these rates could be misleading because of the differences in the testing criteria influencing case identification, hospitalization indications, and case mix across studies (16). Additionally, while some cohorts evaluated in-hospital mortality, others focused on 14- or 28-day survival rates, making comparisons even more problematic. Nevertheless, our rates for the development of critical illness seem somewhat lower than those of most of the studies, as Wiersinga et al. indicate that the overall hospital mortality from COVID-19 is approximately 15%–20% (6).
Hydroxychloroquine was used extensively in our cohort. Whether or not therapeutic modalities affected the mortality rate is beyond the scope of this study (17). Hydroxychloroquine with azithromycin was proposed as a treatment at the beginning of the pandemic based on a small trial (18). However, later well-designed studies failed to show any benefit, and by August 2020, guidelines recommended against their usage (19).
The presenting symptoms in our study were similar to those reported in other studies; cough, dyspnea, and fatigue were the most common (6), and one-fifth of the patients reported anosmia or ageusia (20). Almost all symptoms were more common among patients <60 years than among elderly patients. This might be due to a predilection to hospitalize younger patients with more pronounced symptoms. This finding also suggests that presenting complaints might be subtler among elderly patients, as clinical presentation might vary with age. Sixty-five percent of the patients had at least one comorbidity, such as hypertension, diabetes, or cardiovascular disease, which is consistent with the findings of other studies (6).
Univariable analysis revealed some risk factors for critical illness that were already described in the literature (6, 9, 21, 22). Older age, comorbidities, fever, tachypnea, and hypoxia at presentation were associated with progression to critical illness. Critical illness was also associated with laboratory abnormalities such as lymphopenia, elevated acute phase reactants, and coagulation abnormalities. Contrary to the findings of Zheng et al., smoking status was not associated with developing critical illness (23). This is probably due to a reporting bias, as our cohort’s 4.2% current smoker rate is much lower than the 28.0% daily smoker rate in Turkey (24). While Petrilli et al. found BMI >40 to be independently associated with critical illness development, our findings did not reveal such an association (21). In our cohort, BMI was calculated based on the patient’s self-report and was missing in 67 (14%) participants. Of note, a more recent study analyzing 10,131 cases also did not find obesity and smoking status associated with mortality (25).
Multivariable analysis showed that age was independently associated with developing critical illness, as reported by other studies (6). The number of comorbidities was also associated with critical illness; each comorbidity increased the HR by 1.33. Other cohorts also found the number of comorbidities as a significant predictor for developing critical illness (26). We did not enter specific comorbidities into the model testing, as comorbidities blend and cancel each other out during multivariable analysis. Our model has a high discrimination power with a C-index of 0.92.
The Turkish guidelines for managing COVID-19 recommended hospitalization for COVID-19 patients >50 years, regardless of comorbidity at the beginning of the pandemic (4). However, our analysis showed that for patients <60 years, progression to critical illness is associated with comorbidity. This result suggests that the lower age limit of hospitalization for patients without comorbidity could increase to 60. Such a change would relieve the demand for hospital beds.
Patients with PCT ≥0.25 µg/L and LDH ≥350 U/L had HRs of 2.12 and 2.04, respectively, for critical illness. PCT increases are expected in bacterial infections, suggesting these patients might have coexisting bacterial infections (21). The LDH increase is probably due to cellular damage induced by the virus as well as the immune response against it. PCT and LDH associations were also reported in other studies (21, 27, 28). Although lymphopenia (<800/mm3) and C-reactive protein (CRP) (≥30 mg/dL) remained in the model, they had borderline significance (p=0.05 for both). D-dimer (≥1 mg/L) did not remain in the model. As D-dimer levels correlated with CRP and PCT, it was omitted from the model at the last step of the multivariable analysis.
The WHO scale for clinical improvement was significantly associated with progression to critical illness. Patients on a scale of 4-5 had an HR of 4.15 for critical illness compared to those on a scale of 2-3. The major difference between scales 4-5 and 2-3 is the need for supplemental oxygen. We chose to use the WHO scale in our multivariable model rather than qSOFA because it directly considers the need for oxygen supplementation. Additionally, the WHO scale showed a stronger association with critical illness than the qSOFA score. The WHO scale may reflect the oxygen need more comprehensively as it considers oxygen supplementation directly. In clinical practice, a physician decides to give supplemental oxygen not only for hypoxia but also for dyspnea or tachypnea. Another study from France also found the WHO scale category to be independently associated with developing critical illness (29).
One factor that strongly remained in the model was the hospital admission period. Patients admitted to our hospital at the beginning of May had an HR of 0.14 for progression to critical illness compared to patients admitted at the beginning of the pandemic in mid-March. Such an association was also reported in New York; patients admitted at the beginning of April had an odds ratio (OR) of 0.08 for the risk of critical illness compared to patients admitted at the beginning of March (21). There could be several explanations for this observation. First, our hospitalization criteria did not change as the pandemic progressed; nevertheless, hospital care may have become more efficient with the experience gained in time. Second, up to medication protocols were slightly altered, venous thromboprophylaxis using LMWH was initially administered to patients with classic risk factors for venous thrombosis; then, as coagulopathy became apparent in COVID-19 pathogenesis, more patients hospitalized with suspected or proven COVID-19 received prophylactic LMWH at the beginning of hospitalization unless contraindicated (30). These hypotheses warrant further investigation. Additionally, a less virulent form of the virus might have caused this, as reports show variants of SARS-CoV-2 with differing infectivity and virulence from March to April (31). Furthermore, patients might have been less exposed to the virus due to the increased utilization of preventive and control measures as the pandemic advanced. More recently, a decrease in ICU mortality was also reported between the pre- and post-peak periods of the pandemic (32).
Our study has several limitations. First, although our hospital is one of the main pandemic hospitals within the region, this study is based on a single center’s experience. Second, BMI information was lacking in 14.0% of the participants. Third, we did not quantify the extent of pulmonary involvement in thoracic CT, which might predict the development of critical illness. Finally, our study refers to the beginning of the pandemic and does not consider later developments, such as the emergence of variants, vaccinations, and immunomodulatory and antiviral drugs that have evidently affected the outcome.
In conclusion, age, comorbidity number, the WHO scale, LDH (≥350 U/L), and PCT (≥0.25 µg/L) are independently associated with critical illness development in hospitalized COVID-19 patients. Besides, mortality from COVID-19 seemed to be decreasing as the pandemic advanced. Our findings will be useful for comparison to the other waves of COVID-19.